Data analytics is extremely important for extracting accurate and crucial information out of large mass of data for problem solving and judicious decision making in scientific research. However, the recent transformation in digital technologies has resulted in generating sky rocketing figures- in trillions of gigabytes, of data. But, the inherent complexity of large data sets and their accountability oftentimes is a matter of concern. While there are cultural and technical challenges as well as reservations regarding intelligence of the data, the good news is that most of these can be addressed in an objective way by meaningful experimentation, collaboration, strategy and support to gain actionable insights. Some of the major challenges associated with data analytics and the ways to deal with them are discussed in brief.
1. Data Storage: The exponential increase in the rate of data collection in this data-driven culture is generating an overwhelming amount of data. Storage of such massive amount of data is one of the primary challenges encountered.
There is always more data collected from the experiments than that of which get actually published. These take up a great deal of space. Personally, I had difficulties with my own spectroscopic data storage and handling due to the large size of data files. Also, when you work on several different instruments and want to bring all the data together, there are issues like duplicated data. This also contributes in taking up more storage space.
An automated and intuitive system to screen and organize data would be very beneficial. Also, although local servers provide various advantages like accessibility, security etc. but it gets quite expensive for storing bulk data. Here, cloud storage can enter with a potential solution in reducing cost for data storing while still securing the integrity and reliability of data. And the good news is cloud services are becoming cheaper by day.
2. Skilled Researchers: With the exponential rise in data collection and complexity, we are struggling with their analysis. This is mainly due to the lack of necessary in-depth skill and knowledge of data analysis. There definitely is massive shortage for skilled data analysts in comparison with the voluminous data being generated.
We all normally develop the skill in setting up experimental design and executing those in the particular research practice. In doing so, most of the times, we do not focus on training ourselves with high standards of data analysis tools. But to choose correct statistical tool and make accurate interpretation of the data, scientists need to go beyond just selecting the most suitable statistical model for the data. This is where data analysis becomes a multi-disciplinary. Besides our own research projects, we must get ourselves competent enough to make insightful analysis of the data.
Alternatively, simplifying analysis procedures in terms of technical skills will also help more people to analyse data irrespective of skill level. With customized softwares having user friendly statistical validation and machine learning methods, the computational skills to work with huge data sets can be compromised.
So, at the end it depends whether we upgrade ourselves to explore and format data with complex mathematical models or choose to resort to custom data analysis resources and providers. Whichever way one might mitigate to deal with this issue, ultimate goal will remain same – to analyse, visualize, interpret and present the outcomes of a study in the more informative manner.
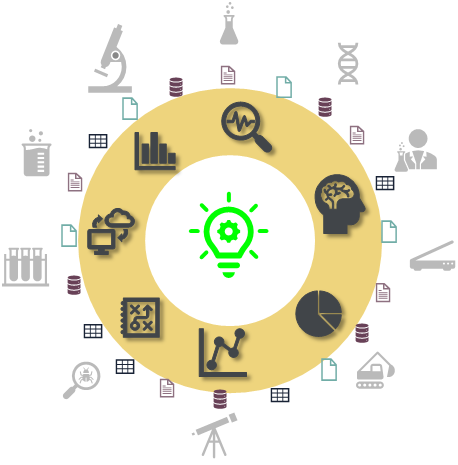
3. Data Cleaning: Once the data is acquired, they need to be sifted through so that an objective analysis can be done with accurate, relevant and consistent set of data. But with such an overwhelming amount of data it gets difficult to clean them manually which might at the end question the integrity of the analysis.
So, to derive accurate and unbiased insight to the data an automatized sophisticated tool using machine learning will be useful. Developing such precise tools which can predict the trends after rigorously cleaning organizing real-time data would definitely lead to more confident and accurate results in short time.
4. Data Visualization: To my understanding data visualization is an amalgamation of science and art. A visually clutter free illustration of data with tools like graphs, charts, bars, histograms, heat maps, scatter plots etc. makes it much easier for human brains to engage in understanding complex data with greater impact.
These visualisation tools help in taking information from real-time data and convert into a visual context. However, these tools aren’t always easy to build manually or use randomly. To select the most befitting tool to an analysis, it is important to take into account some of the criteria like,
- What is the goal of the study
- Who are the audiences
- Which visualisation tool will help detect patterns and outliers of the data set
- Choose the “right” tool accordingly (graph, chart, scatter plot etc.)
- Selecting contrasting colours and well labelled axes is important too
- Include comparisons and references wherever suitable
- Make a clean layout
5. Unified Format: While collecting large volume of data from various sources for different kinds of studies, it is not always possible to record and capture data with same format as different systems use different softwares and many a times data generated are in different formats. This makes it difficult to handle and manage the data while analysing with particular software.
These data from disparate sources need to be synchronized for accurate analysis and to avoid fragmentation of data. Comprehensive algorithms and advanced analytics use these data and process accordingly. A centralized system where the data from different sources can be accessed in one location will also help in cross checking data types thereby reducing potential inconsistencies.
The final goal of data analysis in scientific research is to deliver objective, error-free and authentic data. Besides the above five there are issues like dealing with poor quality of data, dealing with statistical errors, outliers etc. Hence, it is important to try to be diligent and as accurate as possible during data analysis to exact the most reliable information out of them.